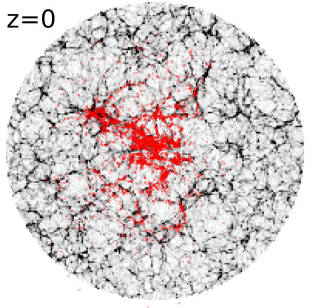
The BORG Particle-Mesh model
We have published a new algorithm that allows to adjust with finely details the structure of the Local Universe from 2M++ data. We present some direct applications to mass measurements, peculiar velocities and density fluctuations.
G. Lavaux, J. Jasche, 2018-07-24
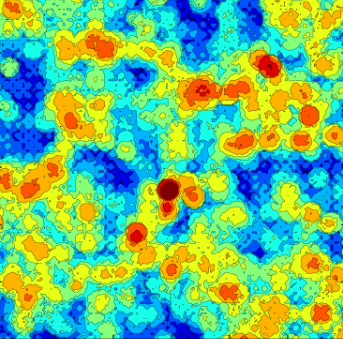
Fifth force on galaxy cluster scale
The tightest bound on fifth-force class of modified gravity models are found on the basis of the BORG-PM model and innovative small scale modelling of the gravitational field. Those bounds even hint at possible positive detection, though more investigation on the impact of galaxy physics is required to assess this claim.
H. Desmond, 2018-08-16
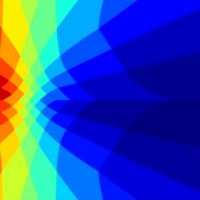
Bayesian treatment of unknown foregrounds
We present an effective solution to the unknown foreground contamination problem in galaxy survey analyses. We have developed a robust likelihood designed to account for effects due to unknown foreground and target contaminations by effectively marginalizing over the unknown large-scale contamination amplitudes.
D. Kodi Ramanah, N. Porqueres, 2018-12-14
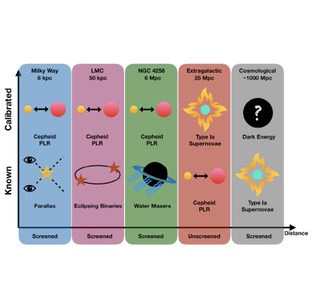
A fifth-force resolution of the Hubble tension
The tension between low and high redshift probes of $H_0$ is possibly the most pressing problem in cosmology today. We explore a novel resolution involving screened fifth forces, which breaks the assumption that gravity behaves identically across rungs of the cosmic distance ladder. Including existing constraints on our models, we are able to reduce the $$H_0$$ tension from 4.4$\sigma$ to 1.5$\sigma$.
H. Desmond, 2019-07-13
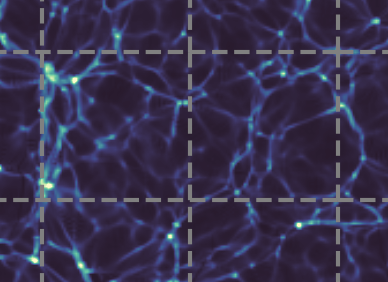
Simulating the Universe on a mobile phone
Existing cosmological simulation methods lack a high degree of parallelism due to the long-range nature of the gravitational force, which limits the size of simulations that can be run at high resolution. In this post, we discuss a new, perfectly parallel algorithm to simulate the Universe on a variety of hardware architectures.
F. Leclercq, G. Lavaux, 2020-05-25
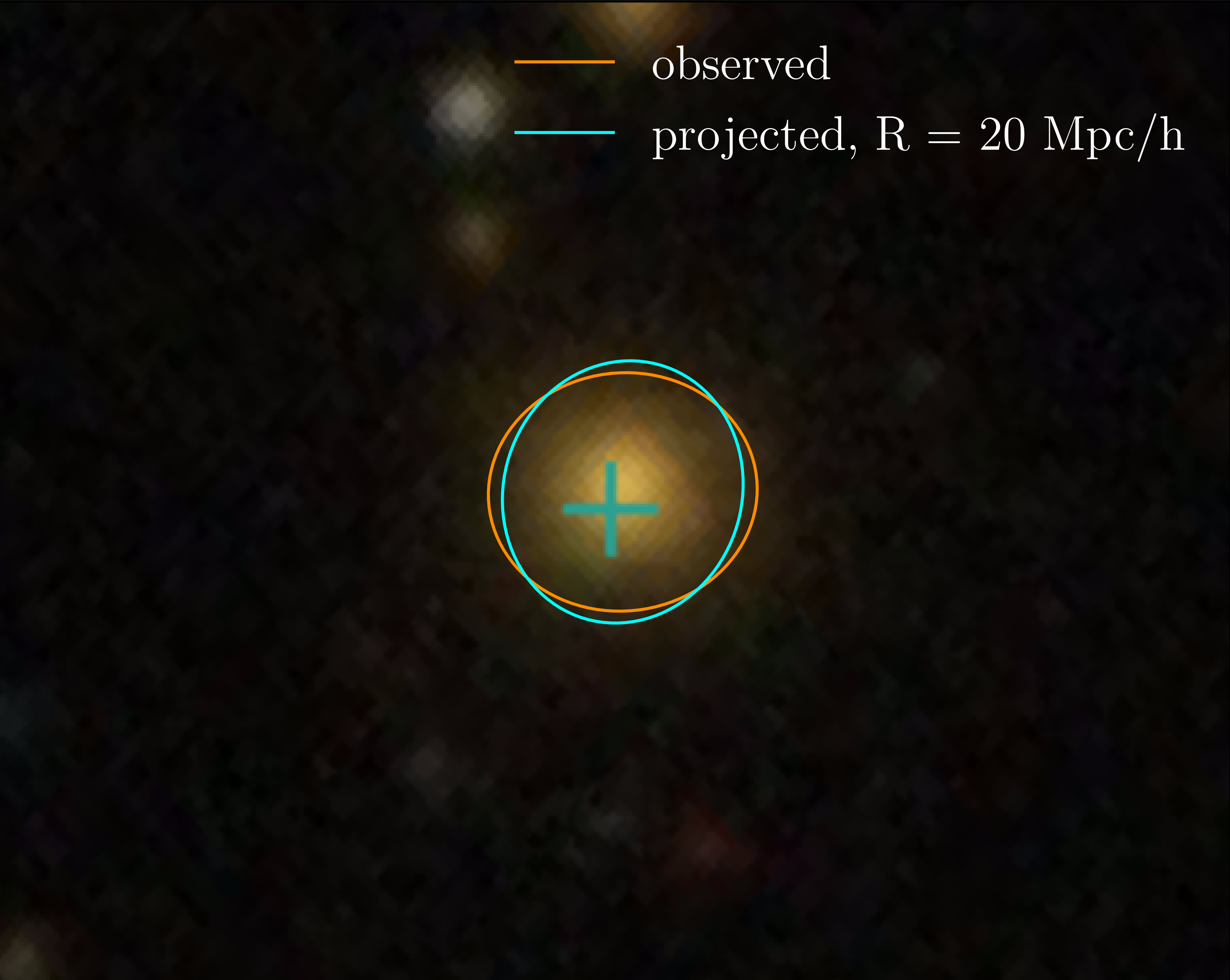
Field-level inference on galaxy intrinsic alignment
Elliptical galaxies tend to align with the large scale structures for two reasons: through intrinsic deformations and tilting during their formation or gravitational weak lensing. Here, we constrain the intrinsic alignment for luminous red giants in the SDSS3-BOSS sample, using 3D tidal fields constrained with forward modeling of SDSS3-BOSS data. We have found 4σ evidence of intrinsic alignment at 20 Mpc/h.
E. Tsaprazi, 2021-12-09
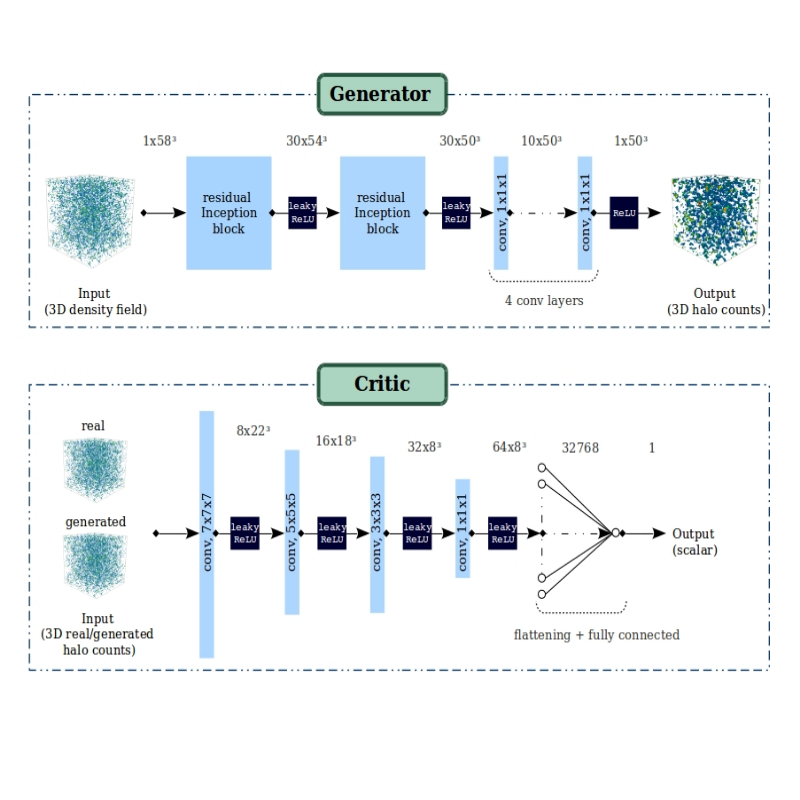
Painting halos from 3D dark matter fields
We present a novel halo painting network that learns to map approximate 3D dark matter fields to realistic halo distributions. We use simple physical principles to train the mapping network to learn the non-trivial local relation between dark matter density field and halo distributions without relying on a physical model.
D. K. Ramanah, 2019-03-31
Neural physical engines for inferring the halo mass distribution function
The tracing of the dark matter distribution by halos is complex and requires the knowledge of unknown small scale astrophysics. We use physically motivated neural networks to agnostically probe this bias model. The tunable parameters of the neural network are inferred as part of the BORG algorithm, and provide an exceptional fit to the halo mass distribution function. No training data is necessary since the network is conditioned on the observed halo catalogue directly.
T. Charnock, 2019-10-15
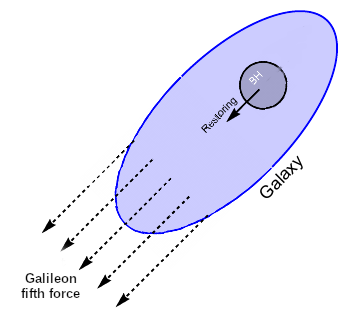
Testing gravity with the positions of supermassive black holes
A key frontier in cosmology is testing the nature of gravity. We use constrained simulations of local structure based on the BORG algorithm to map out the scalar field in galileon gravity, a leading competitor to General Relativity. From this we predict the behaviour of supermassive black holes in galaxies, and then compare with observational data to constrain the coupling strength of the galileon.
H. Desmond, 2020-10-26
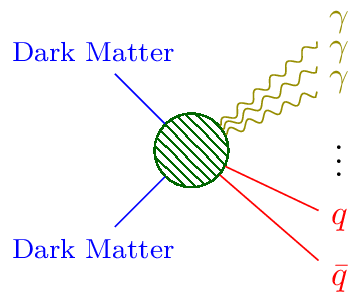
Constraining dark matter annihilation and decay in large-scale structures
The identification of dark matter is a crucial task of modern physics. We present a full-sky, field-level search for dark matter annihilation and decay in the large-scale structure of the nearby universe, exploiting more information than conventional analyses targetting specific objects. We find no evidence for such effects, placing new constraints on the rates of dark matter interactions.
H. Desmond, D. Bartlett, 2022-06-19
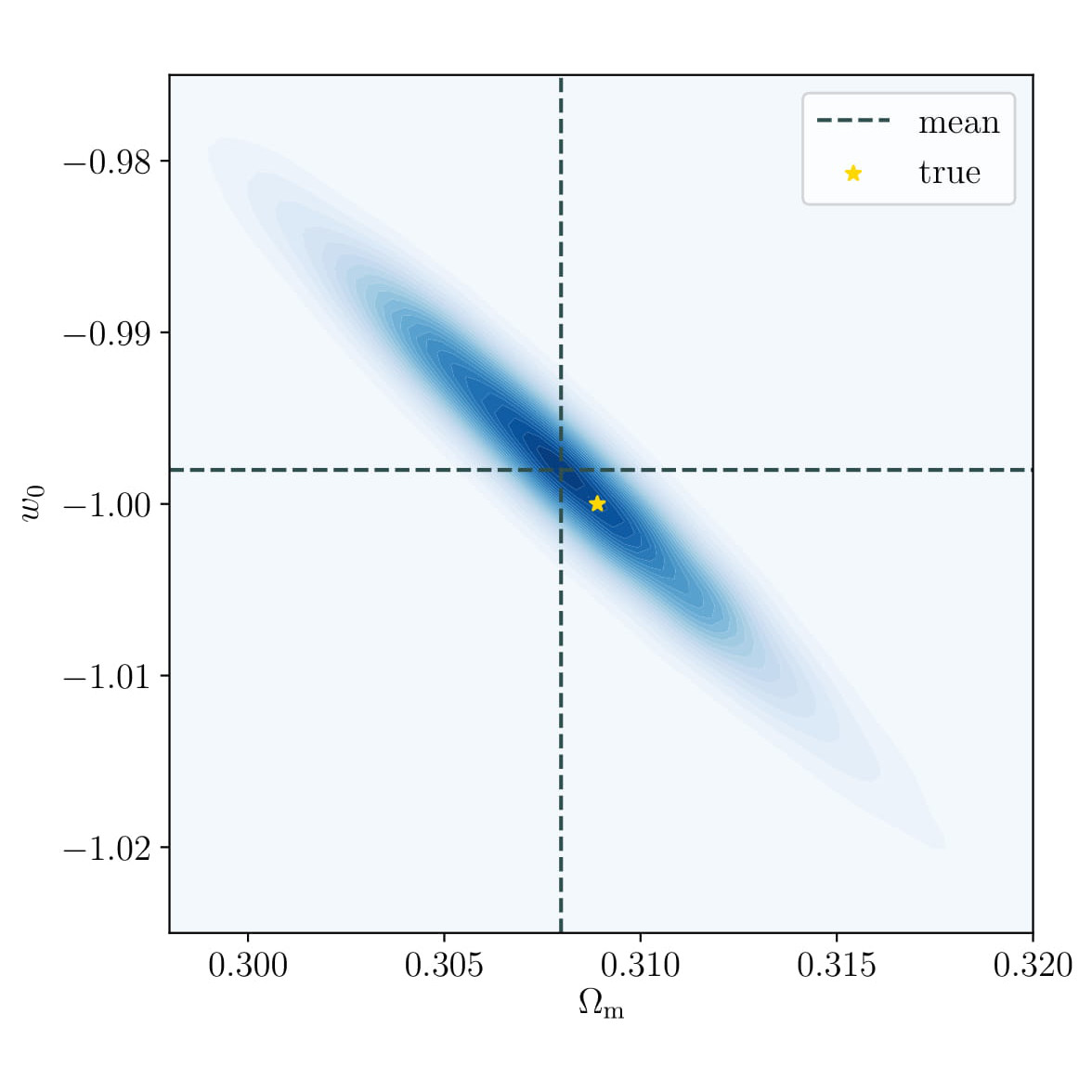
Precision cosmology with expansion
We have developed a novel algorithm to infer cosmological constraints within a large-scale Bayesian inference framework. This hierarchical approach, relying purely on the geometrical symmetries of the cosmological principle, is among the first methods to extract a large fraction of information from statistics other than that of direct density contrast correlations.
D. Kodi Ramanah, G. Lavaux, 2018-08-27
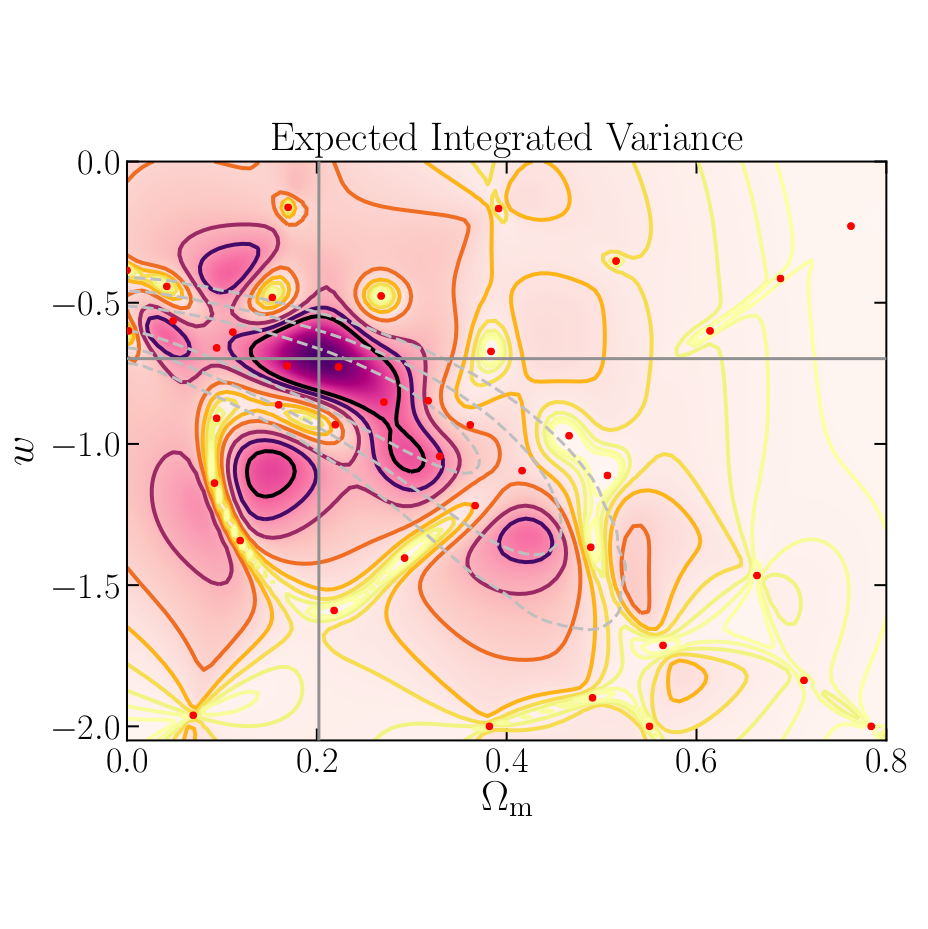
Algorithms for likelihood-free cosmological data analysis
Many numerical models in cosmology can only be simulated forward. We have developed two novel algorithms to perform rigorous statistical inference from these models, in two different scenarios. The first one, BOLFI, reduces the number of required simulations for physical parameter inference by several orders of magnitude. The second one, SELFI, allows a full reconstruction of the primordial matter power spectrum.
F. Leclercq, 2019-04-25
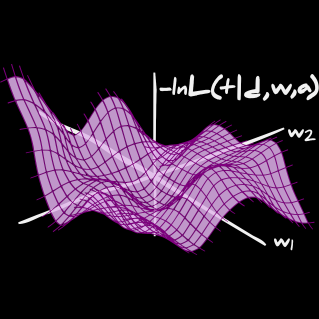
Why neural networks don’t work and how to use them
Throughout the scientific community neural networks are being used for a variety of different tasks. Unfortunately, this is normally done without thought of the statistical implication. Here we lay down the statistical notions showing why neural networks cannot be used by themselves for scientific purposes. We then provide a suite of methods which allows them to be used safely within a statistical framework for parameter inference.
T. Charnock, 2019-12-07
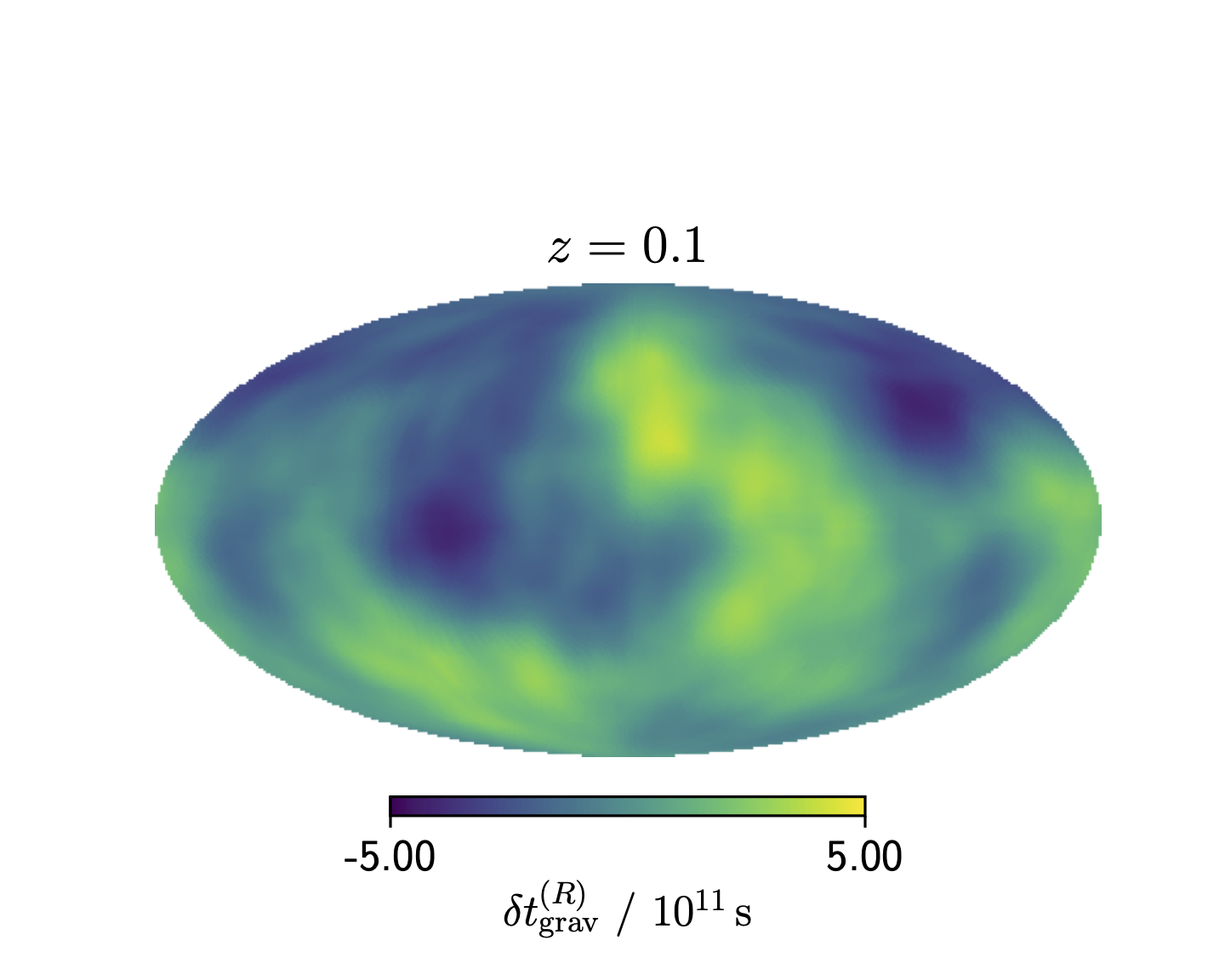
Is the speed of light energy dependent?
High energy astrophysical transients at cosmological distances allow us to test the fundamental assumptions of the standard models of cosmology and particle physics, such as the Weak Equivalence Principle, Lorentz Invariance or the massless nature of the photon. A violation of any of these would result in energy-dependent arrival times for photons from distant sources. We forward model these time delays for gamma ray bursts using the BORG SDSS-III/BOSS reconstruction and compare to data to constrain the quantum gravity energy scale, the mass of the photon, and violations of the Weak Equivalence Principle.
D. Bartlett, 2021-11-06
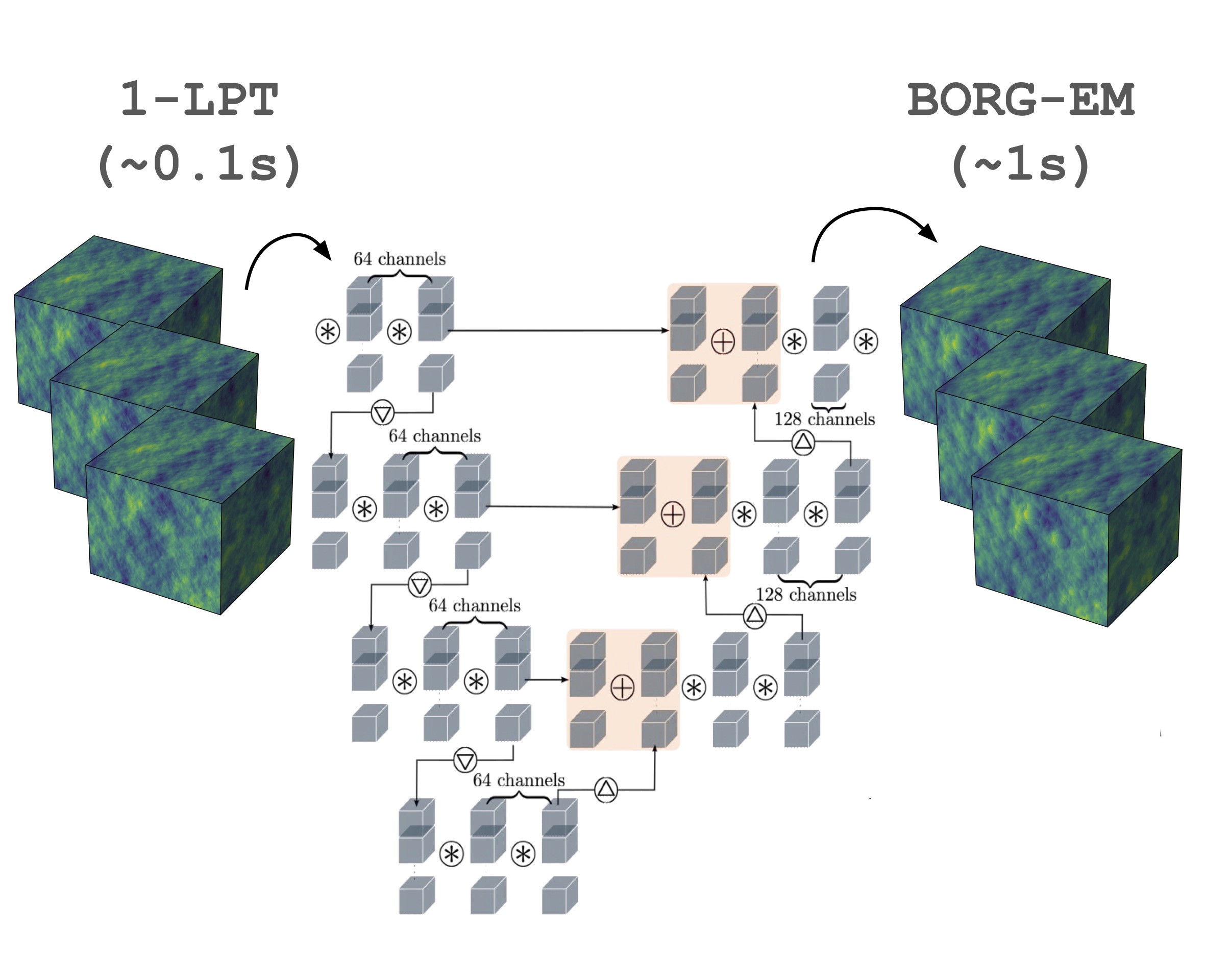
Bayesian Inference of Initial Conditions from Non-Linear Cosmic Structures using Field-Level Emulators
Leveraging a highly accurate physics model essential for next-generation cosmological analysis typically involves significant computational demands. We here propose a solution by integrating a machine learning-based emulator into the BORG algorithm for effective sampling of cosmic initial conditions from non-linear cosmic structures.
L. Doeser, D. Jamieson, S. Stopyra, G. Lavaux, F. Leclercq, J. Jasche, 2023-12-20
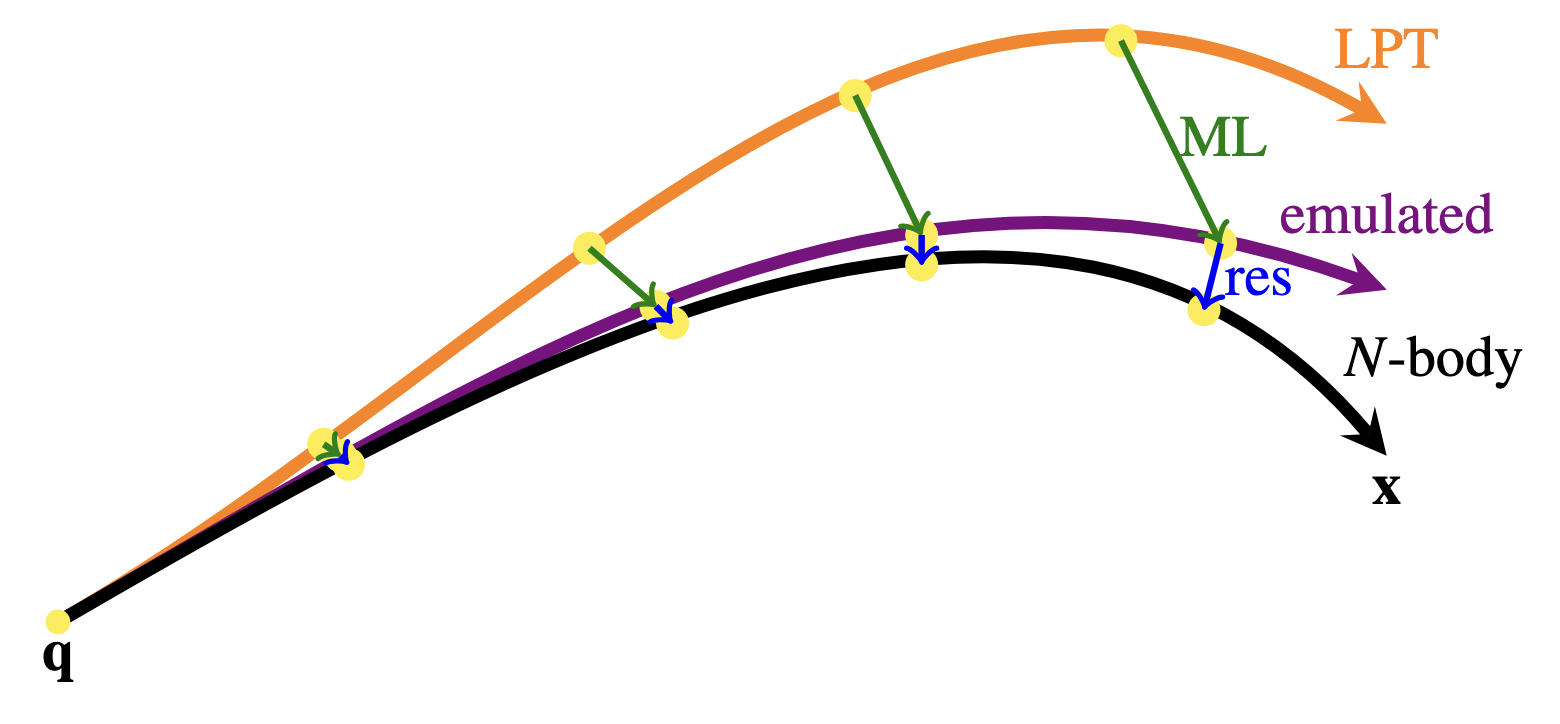
COCA - N-body simulations in an emulated frame of reference
N-body simulations are key to cosmology but are computationally expensive. ML can speed them up, but can we trust the results? We propose a new method of combining ML and simulations (COmoving Computer Acceleration - COCA) which can catch mistakes made by ML and correct for them.
D.J. Bartlett, M. Chiarenza, L. Doeser, F. Leclercq, 2023-01-01